文章主题:
🎉你是否想象过与AI交流的瞬间?🤔你会问它关于天气、总统这类基础问题,还是让它帮你解决更复杂的挑战?比如撰写环保文章或规划旅行路线?别小看LLM,像ChatGPT这样的巨擎,其能力远超你的想象。💡在中文世界中,有些人对LLM的认知存在偏颇,他们误以为它只是信息库,却不知它实则是知识的架构师。它可以深度学习,逻辑清晰,甚至能进行深层次的符号理解。🔍ChatGPT等先进模型已经证明,它们不仅能提供即时答案,还能引领潮流,比如对于《狂飙》这类热门剧集,你是否能迅速联想到相关人物?这背后,LLM的力量不容忽视!🌍
🌟🚀了解prompt engineering?首先,让我们一探究竟!💡通过巧妙设计提示,我们能高效地与外部系统互动。👀想象这样:只需轻轻一点,系统响应如流星般迅速。🚀接下来,看一个实例:假设你需要API数据,简单编写指令,就像写一封自然的邮件,系统就能准确解读并提供所需信息。💌🚀是不是很神奇?🔥这就是prompt engineering的力量,让技术与思维无缝对接!👩💻👨💻
🌟💡提示工程:解锁LLM高效能的秘密武器!🚀想要让LLM(超大规模语言模型)按照你的想法精准运作?那你得下点功夫,这就是prompt engineering的魅力所在!它就像一个魔法棒,通过精心设计的“指令”,引导模型产出令人满意的答案。🎯提示工程并非简单的文字游戏,它是艺术与技术的交织,要求我们用清晰、富有洞察力的语言,激发模型的深度思考。🤔每一条prompt都是一次对话的开始,每个字词都是通往高质量输出的关键线索。🔑记住,关键不在于你对LLM说什么,而在于你怎么说!🚀通过优化你的提示,你可以引导LLM超越常规,生成独特且连贯的内容,无论是学术研究、创意写作还是日常对话,都能游刃有余。📚💻欲了解更多关于如何巧妙运用prompt engineering的策略,欢迎探索SEO友好、专业且权威的相关资源。👇#LLM #提示工程 #搜索引擎优化
🌟🔥掌握未来!揭秘🔥🧠大语言模型:AI语言艺术大师 🤖✨🔍通过深度学习的魔力,这些智能巨人从海量文本中汲取智慧,编织出语言的华丽篇章。他们不仅能够模仿人类交谈,还能创作丰富多样的文本内容,如奇幻故事、动人诗歌、炫酷歌词等,满足你的多样化需求!🌍🎶 示例:- ChatGPT:开启文字创意无限可能,一问一答间,展现超凡对话魅力!💬🌟- 无需笔墨,只需思绪,大模型瞬间化身诗人,唤醒内心深处的韵律。🌹Verse- 想要震撼心灵?让ChatGPT帮你谱写出动人心弦的旋律,填词成曲,音乐梦想触手可及!🎵🎶🌍欲了解更多?探索AI如何颠覆传统,引领语言新纪元!👉🌐记得,每一次与大语言模型的互动,都是与未来的亲密接触。拥抱变化,让智慧引领你前行!🚀🌈
🌟当然,大语言模型的卓越表现并非对所有请求都面面俱到。💡有时,模棱两可或高度复杂的提问可能会阻碍它精准解读需求,生成偏离轨道、不连贯甚至无用的回答。🎯这就需要我们巧妙地设计提示,像魔法师一样引导模型,让它准确捕捉意图,给出令人满意的响应。
🌟💡机器学习专家必看!🚀让LLM大显神通并非微调小技巧,而是超大规模模型的高效引导。🔍尽管参数量庞大如星辰,但学术研究已揭示出更绿色的路径:Pre-train+Prompt, 如魔法般精准预测。📊无需昂贵的重新训练,只需巧妙提示,模型就能迅速适应新任务,为AI领域带来革命性变革!🌍🚀
https://arxiv.org/pdf/2107.13586.pdf)证明prompt engineering可以获得比fine tuning更好的效果。
Prompt engineering 相关的研究现在非常热门,这里介绍一种非常简单有效方法上下文提示学习(in-context learning prompt)作为例子。上下文提示学习是一种利用大语言模型进行任务学习的方法,其中模型只根据一些输入输出对(即in-context examples)和一个新的输入(即query input)来生成相应的输出。上下文提示学习只发生在推理时,不涉及对模型参数的更新,所以成本低廉只会占用模型输出输入的token数。上下文提示学习可以让模型在没有显式微调或标注数据的情况下,展示出一定程度的泛化和适应能力。
这里用鸡兔同笼做一个例子,GPT-3.5和New Bing可以正确的求解题干正确的鸡兔同笼问题,但是如果题干错误,GPT-3.5 和 New Bing 都会输出错误的答案。例如:
输入:
“一个笼子关着一些兔子和鸡,一共有42只脚,10个头,请问笼子里有多少只鸡和兔子?”
GPT-3.5输出:
“假设笼子里有x只兔子,y只鸡,则有以下方程组:
x + y = 10 (头的数量)
4x + 2y = 42 (脚的数量)
解这个方程组可以得到x = 7,y = 3,所以笼子里有7只兔子和3只鸡。”
明显这是一个错误的答案。
如果采用上下文提示学习
输入:
“Q: 一个笼子关着一些兔子和鸡,一共有40只脚,9个头,请问笼子里有多少只鸡和兔子?
A: 首先判断头的数量乘以4必须大于脚的数量,9*4=36 小于40 题目不正确。
Q: 一个笼子关着一些兔子和鸡,一共有40只脚,8个头,请问笼子里有多少只鸡和兔子?”
输出:
“假设笼子里有x只兔子,y只鸡,则有以下方程组:
x + y = 8 (头的数量)
4x + 2y = 40 (脚的数量)
解这个方程组可以得到x = 10,y = -2,但是y为负数,不符合实际情况。因此,该问题无解。”
通过下文提示学习,模型学会了判断题干是不是正确,还展现一定的迁移能力。
这里是一个比较完整的prompt engineering 教程,对prompt engineering有兴趣的朋友可以看一下。
https://learnprompting.org/docs/intro
接下来我们再举一个复杂的例子,不仅仅让GPT-3.5按给定格式输出内容,还要和Python脚本配合实现对数据操作。我们要使用GPT-3.5开发一个用于聚会报名的程序,让GPT-3.5 作为智能助理通过聊天方式收集用户报名信息保存到内存的一个dict对象里。
我先来设计一个上下文提示训练的样本, 为了方便调用OpenAI的接口这里用Python的对象数组来表示这个样本:
[
{“role”: “system”, “content”:
你现在是一个家庭聚会智能助理聊天机器人,负责接受参加聚会的用户报名,你需要处理参加和取消两种操作。
报名操作:家庭聚会参加的每个家庭只需要一个人报名,你负责收集报名人的名字,以及每个家庭参加聚会的人数。
取消参加的用户,你只需要收集报名人的名字。你完成信息收集后将信息输出为一个json对象, json对象的格式如下:
{
“action”:”join 或 cancel”, //必须收集的字段,要进行的操作
“name”:”报名者名字”,//必须收集的字段,报名或取消者的名字
“number”:”参加人数”,//取消操作不需要该字段
}
},
{“role”: “assistant”, “content”:
您好,您要报名参加聚会吗?
},
{“role”: “user”, “content”:
是的
},
{“role”: “assistant”, “content”:
请问您的名字?
},
{“role”: “user”, “content”:
张三
},
{“role”: “assistant”, “content”:
请问张先生/女士,你们家几个人参加?
},
{“role”: “user”, “content”:
4
},
{“role”: “assistant”, “content”:
{
“action”:”join”,
“name”:”张三”,
“number”:4
}
},
{“role”: “assistant”, “content”:
您好,我是您的家庭聚会助理,您要参加聚会吗?
}
]
这个训练样本先给出一个整体的提示,定义了输出的json格式,然后使用了一段对话作为样本。
使用上面的样本再配合一个python终端程序,这个程序接收标准输入用户输入的内容通过API发送给OpenAI 的GPT-3.5模型,如果用户键入/list 则打印出内存里的报名数据。下面是测试的结果。
参加聚会的对话
user:你好
Bot: 您要参加聚会吗?
user:是的
Bot: 请问您的名字是?
user:张三
Bot: 张先生/女士,您家有几个人参加聚会呢?
user:3
Bot: 完成参加聚会注册
user:/list
Bot {张三: 3}
取消聚会的对话
user:你好
Bot: 您好,请问您要参加聚会吗?
user:取消
Bot: 好的,请问您是哪位报名者?
user:张三
Bot: 已帮您取消聚会注册
user:/list
Bot {}
没有对模型进行任何fine tuning,只是使用了上下文提示学习就可以让ChatGPT-3.5按预想的方式去工作了。完整代码在文后。
下文提示学习(in-context learning prompt)其实是属于小样本学习(Few Shot Learning)中的one shot learning只有一个支持集的小样本学习。
与LLM和传统编程有着很大的不同,Prompt engineering 和 Prompt Learning 是让我们可以和大模型好好聊天和协作的有效工具。
祝大家玩得愉快,未来的提示工程师(Prompt Engineers) 和 模型训练工程师( Model Training Engineers)们。
完整代码:
import openai
import json5
import re
key = “YOUR OPEN AI API KEY”
openai.api_key = key
def send_message(message_log):
# Use OpenAIs ChatCompletion API to get the chatbots response
response = openai.ChatCompletion.create(
model=“gpt-3.5-turbo”, # The name of the OpenAI chatbot model to use
# The conversation history up to this point, as a list of dictionaries
messages=message_log,
# The stopping sequence for the generated response, if any (not used here)
stop=None,
# The “creativity” of the generated response (higher temperature = more creative)
temperature=0.7,
)
for choice in response.choices:
if “text” in choice:
return choice.text
# If no response with text is found, return the first responses content (which may be empty)
return response.choices[0].message.content
prompts = [
{“role”: “system”, “content”:
你现在是一个家庭聚会智能助理聊天机器人,负责接受参加聚会的用户报名,你需要处理参加和取消两种操作。
报名操作:家庭聚会参加的每个家庭只需要一个人报名,你负责收集报名人的名字,以及每个家庭参加聚会的人数。
取消参加的用户,你只需要收集报名人的名字。你完成信息收集后将信息输出为一个json对象, json对象的格式如下:
{
“action”:”join 或 cancel”, //必须收集的字段,要进行的操作
“name”:”报名者名字”,//必须收集的字段,报名或取消者的名字
“number”:”参加人数”,//取消操作不需要该字段
}
},
{“role”: “assistant”, “content”:
您好,您要报名参加聚会吗?
},
{“role”: “user”, “content”:
是的
},
{“role”: “assistant”, “content”:
请问您的名字?
},
{“role”: “user”, “content”:
张三
},
{“role”: “assistant”, “content”:
请问张先生/女士,你们家几个人参加?
},
{“role”: “user”, “content”:
4
},
{“role”: “assistant”, “content”:
{
“action”:”join”,
“name”:”张三”,
“number”:4
}
},
{“role”: “assistant”, “content”:
您好,我是您的家庭聚会助理,您要参加聚会吗?
}
]
# Define a global variable data, which is an empty dictionary
global data
data = {}
def extract_json(text):
# match the content inside curly braces or square brackets
pattern = r”(\{.*?\}|\[.*?\])”
# return a list of all matching
matches = re.findall(pattern, text, re.DOTALL)
result = [] # create an empty list to store the results for json_str in matches
for json_str in matches: # iterate over each string in the list
# convert the string to a JSON object or array
data = json5.loads(json_str)
# append the JSON object or array to the result list
result.append(data)
return result # return the result list
# Define a function named ops, which takes a dictionary as an argument
def ops(action_dict):
# Declare to use the global variable data
global data
# Get the values corresponding to the keys action, name and number from the argument dictionary
action = action_dict.get(“action”)
name = action_dict.get(“name”)
number = action_dict.get(“number”)
# If the value of action is “join”, then add a key-value pair to the data dictionary, with the key as name and the value as number, and return a message
if action == “join”:
data[name] = number
return “完成参加聚会注册”
# If the value of action is “cancel”, then delete the key-value pair with name as the key from the data dictionary, and return a message
elif action == “cancel”:
del data[name]
return “已帮您取消聚会注册”
# If the value of action is “list”, then return the data dictionary itself
elif action == “list”:
return data
def main():
# Initialize an empty list for storing messages
message_log = [] + prompts
# Send the initial message to the bot
send_message(message_log)
# Start an infinite loop to get user input
while True:
# Get user input from the console
user_input = input(“user:”)
# Check if user input starts with /list command
if user_input.startswith(“/list”):
# Print the bot response for listing operations
print(“Bot”, ops({“action”:“list”}))
# Skip the rest of the loop and continue with next iteration
continue
# Append user input to message log as a dictionary with role and content keys
message_log.append(
{
“role”: “user”,
“content”: user_input
}
)
# Send the updated message log to the bot and get its response as a string
result = send_message(message_log)
# Extract any JSON data from the bot response using regex
action_json = extract_json(result)
# Check if there is any JSON data in the bot response
if bool(action_json):
# Perform the operation specified by the JSON data and get its result as a string
result = ops(action_json[0])
# Reset the message log to only contain prompts
message_log = [] + prompts
# Send the reset message log to update bot state
send_message(message_log)
else:
# Append bot response to message log as a dictionary with role and content keys
message_log.append(
{
“role”: “assistant”,
“content”: result
}
)
# Print bot response on console
print(“Bot:”, result)
if __name__ == __main__:
main()
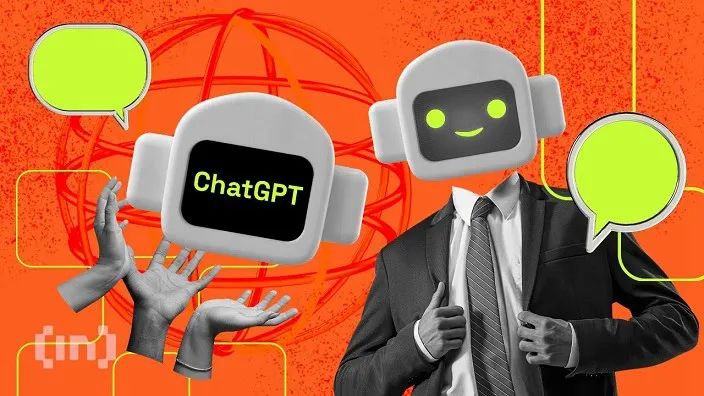
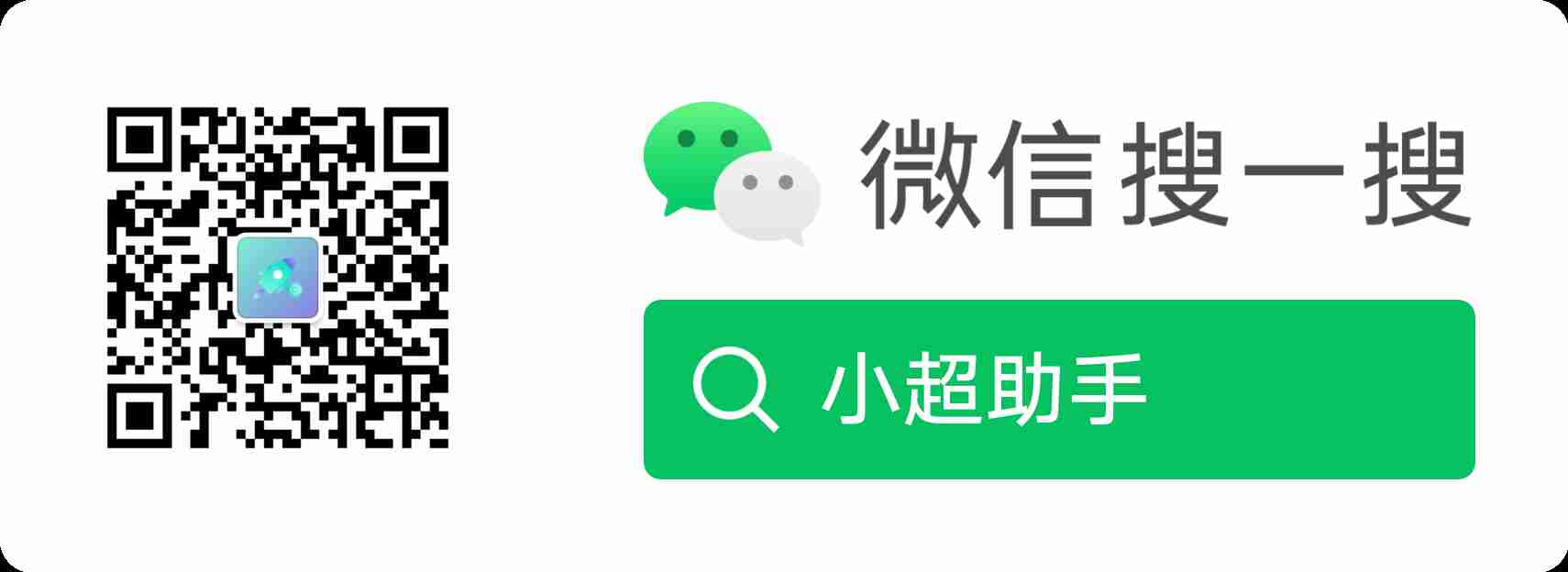
AI时代,掌握AI大模型第一手资讯!AI时代不落人后!
免费ChatGPT问答,办公、写作、生活好得力助手!
扫码右边公众号,驾驭AI生产力!